Remote Sensing, Free Full-Text
Por um escritor misterioso
Last updated 30 outubro 2024
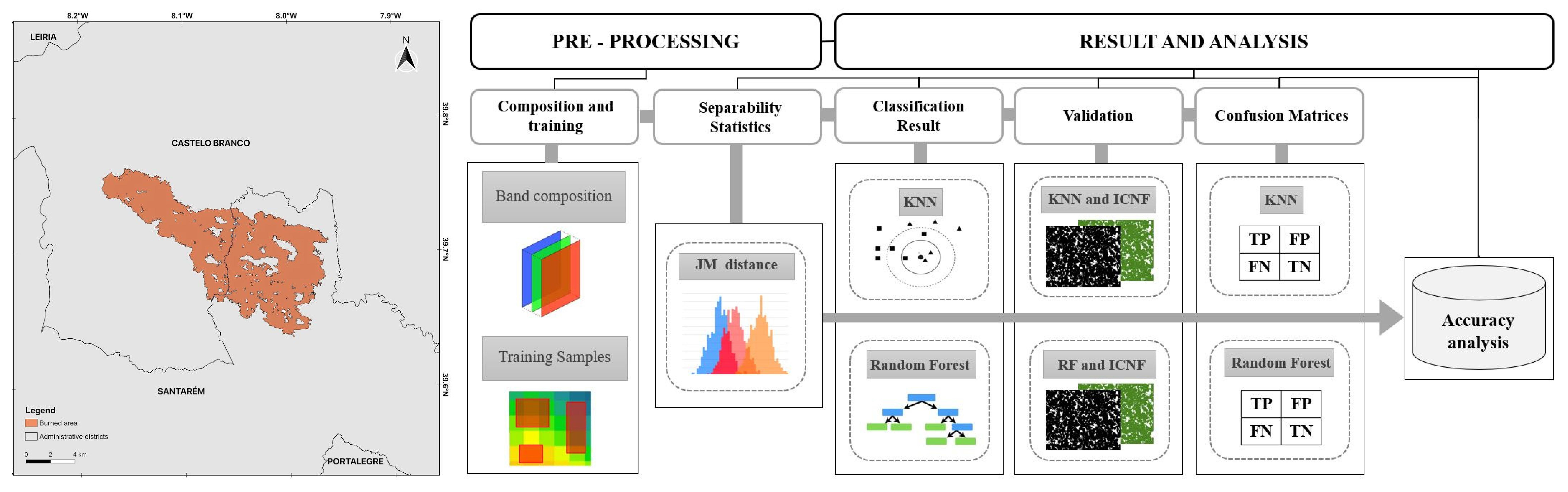
Forest fires threaten the population’s health, biomass, and biodiversity, intensifying the desertification processes and causing temporary damage to conservation areas. Remote sensing has been used to detect, map, and monitor areas that are affected by forest fires due to the fact that the different areas burned by a fire have similar spectral characteristics. This study analyzes the performance of the k-Nearest Neighbor (kNN) and Random Forest (RF) classifiers for the classification of an area that is affected by fires in central Portugal. For that, image data from Landsat-8, Sentinel-2, and Terra satellites and the peculiarities of each of these platforms with the support of Jeffries–Matusita (JM) separability statistics were analyzed. The event under study was a 93.40 km2 fire that occurred on 20 July 2019 and was located in the districts of Santarém and Castelo Branco. The results showed that the problems of spectral mixing, registration date, and those associated with the spatial resolution of the sensors were the main factors that led to commission errors with variation between 1% and 15.7% and omission errors between 8.8% and 20%. The classifiers, which performed well, were assessed using the receiver operating characteristic (ROC) curve method, generating maps that were compared based on the areas under the curves (AUC). All of the AUC were greater than 0.88 and the Overall Accuracy (OA) ranged from 89 to 93%. The classification methods that were based on the kNN and RF algorithms showed satisfactory results.
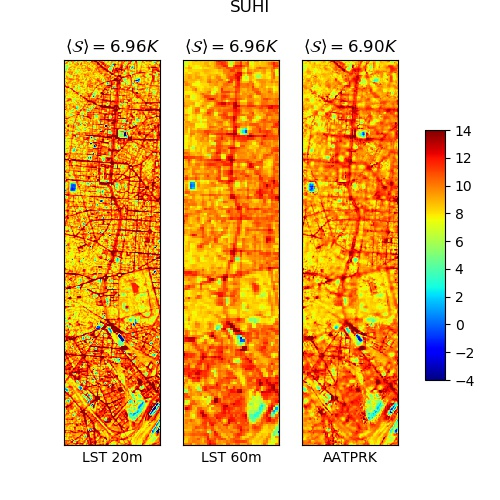
Dota Map 6.85K Free - Colaboratory

Remote Sensing, Free Full-Text, Neural Network Based Quality Control of CYGNSS Wind Retrieval, HTML
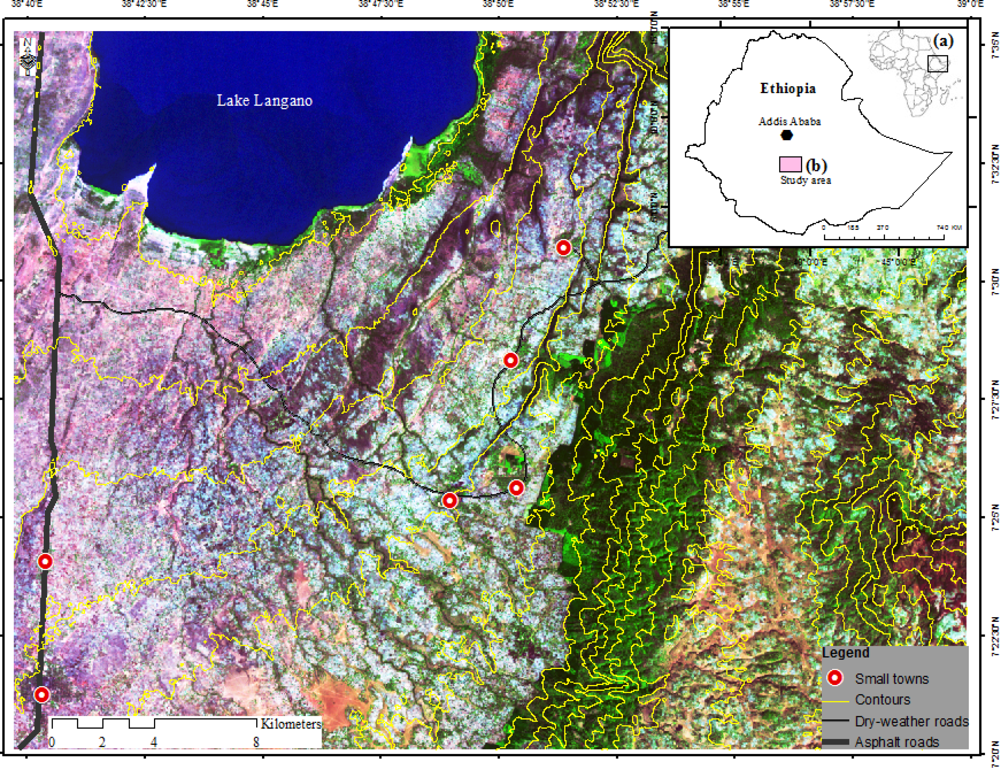
Remote Sensing, Free Full-Text

Remote Sensing and Image Interpretation by Thomas M. Lillesand
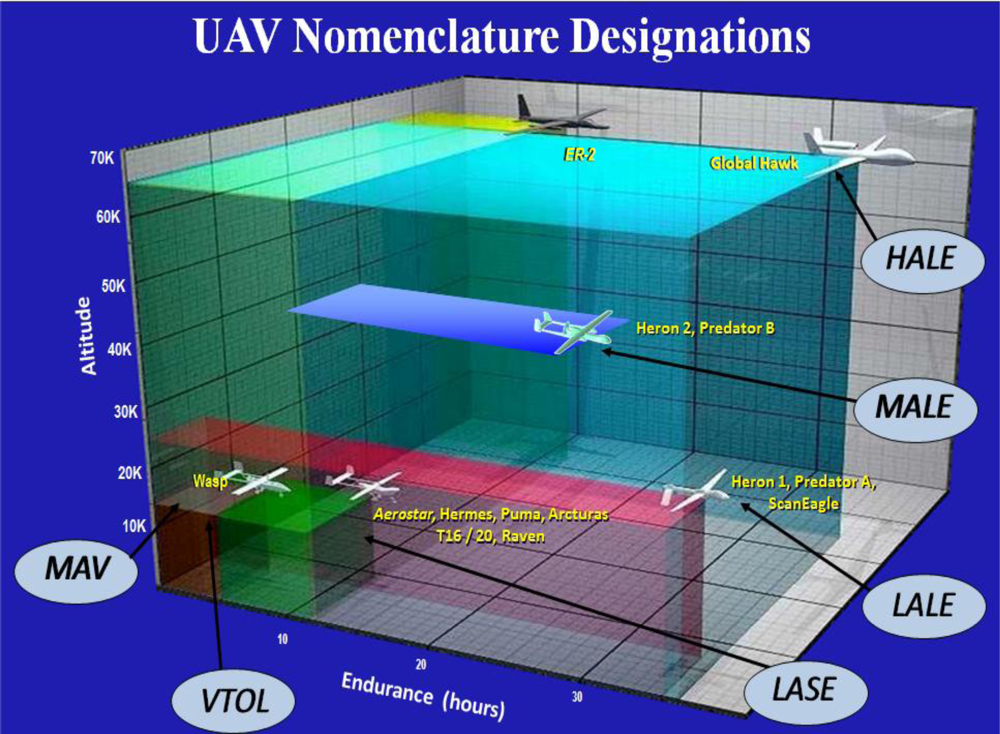
Remote Sensing, Free Full-Text
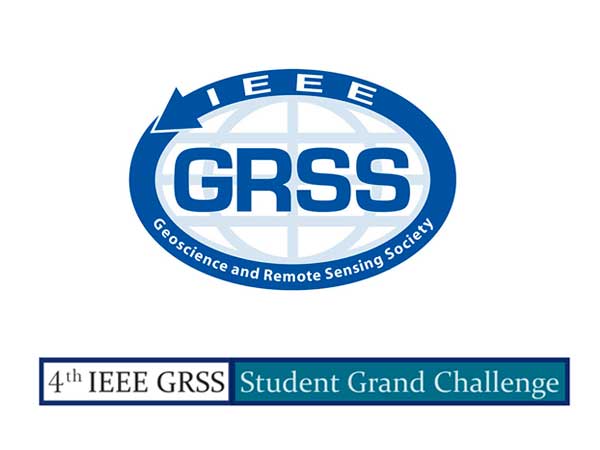
Homepage - GRSS-IEEE
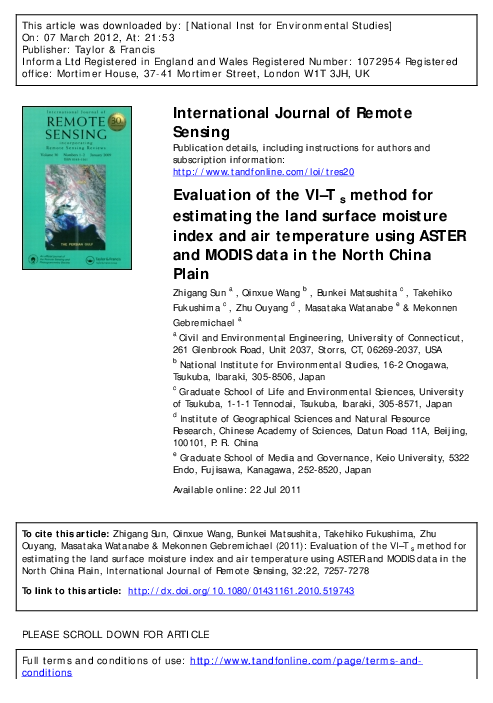
PDF) Assessing potential of MODIS derived temperature/vegetation condition index (TVDI) to infer soil moisture status
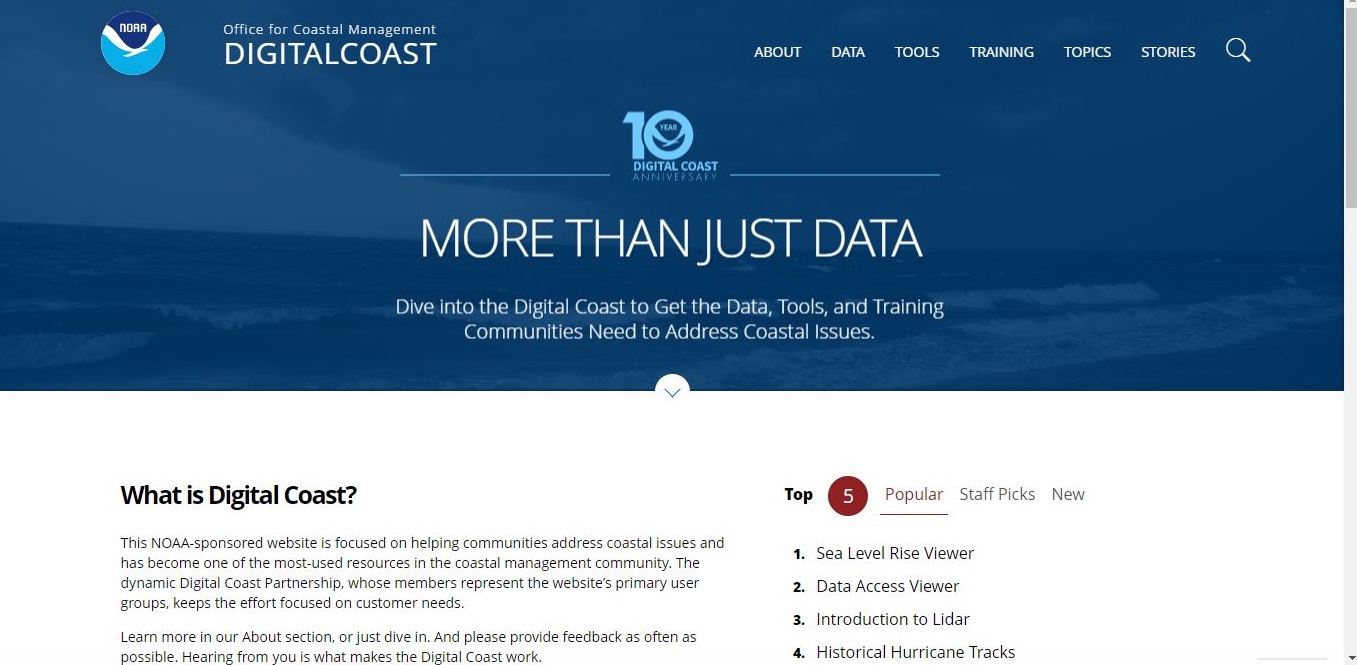
List of Top 10 Sources of Free Remote Sensing Data - Geoawesomeness

PDF) Integrating geographical information systems, remote sensing, and machine learning techniques to monitor urban expansion: an application to Luanda, Angola
Remote sensing of the environment : an earth resource perspective : Jensen, John R., 1949- : Free Download, Borrow, and Streaming : Internet Archive
Recomendado para você
-
91xE7b6lfEL._CLa, 2387,1300, 71nLa7gaL6L.jpg,81qlgE0uKwS.jpg30 outubro 2024
-
Renato Falcão Dantas30 outubro 2024
-
Graphene-Type Materials for the Dispersive Solid-Phase Extraction Step in the QuEChERS Method for the Extraction of Brominated Flame Retardants from Capsicum Cultivars30 outubro 2024
-
510kRwERo1L._CLa, 597,500, 51k1tROyz9L.jpg,51zWZl8ZIhL.jpg30 outubro 2024
-
Int. J. Environ. Res. Public Health, Volume 20, Issue 5 (March-1 2023) – 901 articles30 outubro 2024
-
Direito regulatório - Casa do Direito30 outubro 2024
-
Wine Store in Virginia Beach, VA 23454 - Yiannis Wine Shop30 outubro 2024
-
Renato Braz se eleva em álbum que reúne Chico Buarque, Gilberto Gil e Milton Nascimento, Blog do Mauro Ferreira30 outubro 2024
-
Locke & Key (TV Series 2020–2022) - “Visual Effects by” credits - IMDb30 outubro 2024
-
Laredo United High School class of 2023 graduates30 outubro 2024
você pode gostar
-
Kotoura-San Vol 3 (Shojo Manga) See more30 outubro 2024
-
oreshura.wikia.com - Ore no Kanojo to Osananajimi g - Ore Shura Wiki A30 outubro 2024
-
Step Back Roblox ID - Roblox music codes30 outubro 2024
-
Editor de Vídeos em 4K - Kizoa30 outubro 2024
-
Sword Race Codes Wiki Roblox [NEW] [November 2023] - MrGuider30 outubro 2024
-
Popular anime series 'DanMachi' gets mobile game by Crunchyroll30 outubro 2024
-
Dave Bautista, Knives Out Wiki30 outubro 2024
-
Vanitas, Pandora Hearts Wiki, Fandom30 outubro 2024
-
Kankuro - Boruto render by Sispros on DeviantArt30 outubro 2024
-
900+ ideias de Caminhoes de todas as marcas e modelos30 outubro 2024